Subsections of Dictionaries
The Dictionary<TKey, TValue> Class
The Dictionary<TKey, TValue> Class
In this section, we will discuss the use of the Dictionary<TKey, TValue>
class, which implements a dictionary. In the next
section, we
will discuss how this data structure can be implemented using a linked
list. In subsequent sections, we will consider alternative
implementations.
Note that the Dictionary<TKey, TValue> type has two type parameters,
TKey and TValue. TKey is the type of the keys, and
TValue is the type of the values (i.e., the data elements associated
with the keys). Keys must always be non-null — any attempt to use a
null key will result in an ArgumentNullException. A
Dictionary<TKey, TValue>’s most basic public methods are:
Note
The type of the value
parameter for TryGetValue is actually TValue, not TValue?. Another kind of annotation is used to indicate that this out parameter may be null only when the method returns false. Because such annotations are beyond the scope of CIS 300, we will treat this parameter as if it were simply defined as being nullable.
The above methods can be used for building and updating a
Dictionary, as well as for looking up values by their keys. It is
also possible to do updates and lookups using indexing. Specifically, a
key may be used as an index in a Dictionary, as if the
Dictionary were an array. For example, suppose that dictionary
is
a Dictionary<TKey, TValue>, k
is a TKey, and v
is a
TValue. We can then do the following:
This will associate the value v
with the key k
, as the Add
method does; however, its behavior is slightly different if k
already
has a value associated with it. Whereas the Add method would throw
an exception in this case, using the indexer will simply replace the
value previously associated with k
by the new value v
. Thus, we use
the Add method when we expect the key to be a new key for the
dictionary, but we use the indexer when we want to associate the value
with the key, regardless of whether the key is already in the
dictionary.
Likewise, we can use the indexer to look up a key:
Again, the behavior is similar to the TryGetValue method, but
slightly different, as there is no bool in the above statement. When
using the indexer, if the key is not in the dictionary, it will throw a
KeyNotFoundException. Thus, we use the indexer when we expect the
key to be in the dictionary, but we use the TryGetValue method when
we don’t know if the key is in the dictionary.
Implementing a Dictionary with a Linked List
Implementing a Dictionary with a Linked List
One way of implementing a dictionary is to store all the keys and values
in a linked list. We want to do this in such a way that a key is stored
together with its associated value. To facilitate this, .NET
provides a structure
KeyValuePair<TKey, TValue>
in the System.Collections.Generic namespace. This structure is used
simply for storing a key and a value. The type parameter TKey is
used to define the type of the keys, and the other type parameter
TValue is used to define the type of the values. It has two
public properties:
- Key,
which gets the key stored; and
- Value,
which gets the value stored.
Note that neither of these properties can be set; i.e., the structure is
immutable. In order to set the key and value, we need to construct a new
instance using its 2-parameter
constructor.
The first parameter to this constructor is the key, and the second is
the value.
Now that we have a way of storing keys and values together, we can
implement a Dictionary<TKey, TValue> with a linked list
comprised of instances of
LinkedListCell<KeyValuePair<TKey, TValue>>. Thus, each
cell of the list stores as its Data a
KeyValuePair<TKey, TValue> containing a key and its
associated value. To add a key and a value, we first need to search the
list for a cell containing that key. If we find such a cell, we either
replace the KeyValuePair in that cell with a new KeyValuePair
containing the given key and value, or we throw an exception, depending
on the specific behavior required. If we don’t find such a cell, we
insert a new cell containing the given key and value. Because it doesn’t
matter where we insert it, we might as well insert it at the beginning
of the list, as that is the easiest way. We can remove a key using
techniques described in “Finding Prime
Numbers”.
The main disadvantage to this approach is that searching for a key is
expensive. For example, to search for a key that is not in the
dictionary, we need to examine every key in the dictionary. We would
like to improve on this performance.
One way of improving the performance of searching is to store the keys
in increasing order. Then as we search, if we see a key that is larger
than the key we are looking for, we can stop. However, recall that keys
can be of any type. For some types of keys, “increasing order” and
“larger than” make no sense.
C# does provide a way to restrict the types
that can be passed as type parameters to generic types. Specifically, we
can restrict the type TKey by writing the class statement as
follows:
public class Dictionary<TKey, TValue> where TKey : notnull, IComparable<TKey>
The where clause in this statement constrains TKey in two ways:
notnull
constrains it to be a non-nullable type. The compiler doesn’t actually enforce this constraint, but will give a warning if a nullable type is used for TKey.
IComparable<TKey>
constrains it to be a
subtype of
IComparable<TKey>.
Each subtype of IComparable<TKey> contains a method public int
CompareTo(TKey?
x). If a
and b
are of type TKey, then a.CompareTo(b)
returns:
- A negative number if
a
is considered to be less than b
; - 0 if
a
is considered to be equal to b
; or - A positive number if
a
is considered to be greater than b
or if b
is null.
We can therefore use this CompareTo method to keep the list in
increasing order.
Note that by constraining the key type in this way, we are making the
Dictionary<TKey, TValue> less general, as we may sometimes want
to use a key type that can’t be ordered. On the other hand, there are
times when not only do we have a key type that can be ordered, but also
we need to access the keys in increasing order (for example, to print an
ordered list of keys with their values). In such cases, what we actually
need is an ordered dictionary, which both restricts the keys in this
way and provides a means of accessing them in increasing order. While we
won’t consider the full implementation of an ordered dictionary here, it
is worth considering how we can improve performance by keeping the keys
in increasing order.
Let’s now consider how to add a key and value to a linked list storing
keys in increasing order. We first need to find where the key belongs in
the ordering. Specifically, the cell whose Next property needs to be
changed (assuming the key is not already in the list) is the one that
must precede the new cell. We therefore need to find the last cell whose
key is less than the key we need to add. Note also that when we are
removing a key, the cell whose Next property needs to be changed is
the last cell whose key is less than the key we are removing.
Furthermore, if we are looking up a key, we need to look in the cell
that follows the last cell whose key is less than the key we are looking
for. This suggests that we should provide a private method to find
the last cell whose key is less than a given key, provided such a cell exists.
Before we can write such a method, however, we first need to address a
problem that occurs if we are trying to add, remove, or look up a key
that is smaller than all other keys in the list. In this case, there are
no cells containing keys smaller than the given key.
We can avoid needing a special case to deal with this problem if we
include a special header cell at the beginning of our linked list.
This cell will not contain any meaningful data, but it will always be
present. If we consider that its key is less than any other key (though
we will never actually examine its key), then there will always be at
least one key less than any given key. We can obtain this
header cell by initializing the linked list to contain a new cell containing the default key-value pair,
rather than to null. Note that because the linked list will always contain at least the header cell, the reference to it should not be nullable.
Warning
Setting the data in the header cell to the default key-value pair means that if the key type and/or the value type is a reference type, then it will be null in this pair, even if the type isn’t nullable. There is no way to avoid this, as the only key and value objects that we know of are the default values, which may be null. However, it doesn’t make sense to use KeyValuePair<TKey?, TValue?> as the type of the data items within the linked list just because of the header cell, whose data we don’t intend to use. Furthermore, the compiler won’t generate a warning when KeyValuePair<TKey, TValue> is used. We should therefore use this latter type, and be sure not to use the data stored in the header cell. We should also include a warning of possible null values in a comment when we initialize the header cell.
A method to find the last cell containing a key less than a given key is
now straightforward. We initialize a variable to the first cell (i.e.,
the header cell), and set up a loop that iterates as long as the next
cell is non-null and contains a key less than the given key. Each
iteration advances to the next cell. When the loop terminates, we return
the cell we have reached.
To look up a key, we use the above method to find the last cell
containing a key less than the key we are looking for. If the next cell
is non-null and contains the key we are looking for, then we have
found it; otherwise, it cannot be in the list. To add a key and value,
we first need to look up the key in the same way. If we don’t find it,
we insert a new cell containing this key and value following the last
cell containing a key less than this key. To remove a key, we proceed in
a similar way, but if we find the key, we remove this cell from the
list.
While keeping the keys in increasing order improves the performance of
many searches, the overall performance is still unsatisfactory for even
data sets of moderate size. In subsequent sections, we will explore ways
of improving this performance using various data structures.
Implementing a Dictionary with an Array-Like Structure
Implementing a Dictionary with an Array-Like Structure
In the previous
section,
we discussed how linked lists could be used to implement a dictionary.
An alternative to a linked list would be an array. A couple of other
alternatives are the non-generic
System.Collections.ArrayList
or the generic
System.Collections.Generic.List<T>.
These classes are similar to singly-dimensioned arrays, but they can
grow as needed. In this respect, they are like a
StringBuilder,
but instead of storing chars, an ArrayList stores object?s
and a List<T> stores instances of the type parameter T.
Elements can be retrieved from instances of these classes using
indexing, just like retrieving an element from an array.
Assuming we restrict the keys to be non-nullable sub-types of
IComparable<TKey>, where TKey is the key type, we can store
the keys in order in any of these data structures. We can then search
for a key in the same way as we described for a linked list. However,
such a search can be expensive - to search for a key that is larger than
any key in the dictionary, we need to examine all of the keys. We say
that the performance of this sequential search is in
$ O(n) $, where
$ n $
is the number of keys in the dictionary. This means that as
$ n $ grows,
the time required for the search is at worst proportional to
$ n $.
We can improve this performance dramatically for an array or array-like
structure such as an ArrayList or a List<T> using a technique
called binary search (there isn’t much we can do to improve the
performance of searching a linked list, as its structure restricts us to
traversing it sequentially). The idea is similar to what humans do when
looking for something in an ordered list such as a dictionary or an
index of a book. Rather than looking sequentially through the sequence,
we first look in the middle and narrow our search space depending on how
what we are looking for compares with what we are looking at. For
example, if we are looking for “Les Miserables”, we first look in the
middle of the sequence, where we might see “Othello”. Because “Les
Miserables” is alphabetically less than “Othello”, we can narrow the
search space to those titles less than “Othello”. In the middle of this
search space, we might find the title, “Great Expectations”. Because
“Les Miserables” is alphabetically greater than “Great Expectations”, we
narrow the search space to those titles greater than “Great
Expectations” and less than “Othello”. We continue narrowing in this way
until either we find “Les Miserables” or the search space becomes empty,
implying that the data set does not contain this title.
In a binary search, each lookup is as nearly as possible in the center
of the search space. This means that each time we look at an entry, we
either find what we are looking for, or we decrease the size of the
search space to at most half its previous size. For large data sets the
search space therefore shrinks rapidly. For example, if we start with
1,000,000 elements and repeatedly reduce the search space to at most
half its previous size, after 20 such reductions, we are left with
nothing. Likewise, if we start with 1,000,000,000 elements, 30 such
reductions in size lead to an empty search space.
To implement this algorithm, we need to keep track of the search space.
We will use two int variables, start
and end
. start
will keep
track of the first index in the search space, while end
will keep
track of the first index past the search space, as follows:
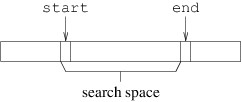
The way we have defined end
may seem unnatural at first, but because
it simplifies various calculations, it is a common way of describing a
search space. For example, the number of elements in such a search space
is simply the difference between end
and start
, and to describe an
entire array, we can initialize start
to 0 and end
to the array’s
length.
We then need a loop to iterate as long as this search space is nonempty
(we can return from inside this loop if we find what we are looking
for). On each iteration, we need to find the midpoint of the search
space. This midpoint is simply the average of start
and end
- i.e.,
their sum divided by 2. We need to be a bit careful here because we are
doing integer division, which may involve truncation. As a result, we may
not get exactly the average. In any case, we need to ensure that the
index we compute is within the search space - otherwise, we may not
reduce the search space, and an infinite loop will result. Because the
search space is nonempty, start
< end
; hence, the true
average is strictly between start
and end
. If this average is not an
integer, the result will be rounded down to the next smaller integer.
Because start
is an integer, this result will be no less than start
,
but less than end
; hence it will be in the search space.
Once we have computed this midpoint, we need to compare the key of the
element at that location with the key we are looking for. Recall that we
use the CompareTo method to do this comparison. Note that for large
key types, the CompareTo method can be expensive. For this reason,
it is best to call the CompareTo method only once for a given pair
of keys, and if necessary, save the result it returns in order to make
more than one comparison between this result and 0.
Thus, once we have obtained the result of the CompareTo method, we
need to determine which of the three cases we have. If the keys are
equal, we should be able to return. If the key we are looking for is
less than the key at the midpoint, we need to adjust end
. Otherwise,
we need to adjust start
. We are then ready for the next iteration of
the loop.
If the loop finishes without returning, then the search space is empty;
hence, the key we are looking for is not in the data set. However,
start
will end up at the point at which this key could be inserted;
hence, the binary search can be used for both lookups and insertions.
Binary search is a very efficient way to search an ordered array-like
structure. In particular, it always makes no more than
$ O(\log n) $
comparisons, where
$ n $ is the number of elements in the data set. The
$ \log $ function grows very slowly - much more slowly than
$ n $.